The Rapid Evolution of AI: Why It Matters for DAM and Content Operations
The Artificial Intelligence (AI) landscape is evolving at an unprecedented pace. 73% of CIOs and executives surveyed by Gartner in 2024 said they were increasing their funding for AI. With so much happening, news broadcasters might soon need a dedicated AI news desk to track developments. Just in the past weeks:
- The US government announced a $500B AI fund
- OpenAI launched Operator
- DeepSeek introduced the R1 model
- Talks of a global “battle” for AI dominance are escalating
For professionals in Digital Asset Management (DAM), content operations, and marketing, these advancements present both opportunities and challenges. Businesses must assess how best to integrate AI agents into their workflows to scale content operations efficiently, manage the explosion of digital assets, and deliver personalized, real-time experiences that align with evolving customer expectations. Implementing AI agents thoughtfully can unlock new levels of efficiency, reduce operational costs, and deliver deeper insights, ultimately driving business agility and competitive advantage.
2025: The Year of AI Agents
We believe 2025 will be the year of AI agents.
Unlike traditional AI models that primarily generate content or analyze data, AI agents function as autonomous assistants, actively interacting with systems, making real-time decisions, and executing tasks without human intervention. This shift has profound implications for businesses seeking to optimize workflows, reduce manual labor, and improve overall operational efficiency.
In this blog, we will explore the role of AI agents, how they differ from generative AI, and the transformative impact they will have on DAM systems and content operations.
What are AI Agents?
An AI agent (also referred to as Agentic AI) is an intelligent system designed to perform tasks autonomously, often in response to dynamic inputs or changing environments. These agents are built with the ability to perceive their surroundings, make decisions based on the information they gather, and then execute actions without requiring constant human oversight. Essentially, an AI agent can interact with other software, modules, or systems, learn from the environment, and improve its decision-making capabilities over time.
In content operations, AI agents can be thought of as digital workers that handle routine tasks such as content tagging, sorting, and even content creation. These agents can continuously improve their performance by analyzing data and receiving feedback. For example, an AI agent working in a Digital Asset Management (DAM) system could automatically categorize new content based on pre-established guidelines, saving time and reducing human error.
How are AI Agents Different from Generative AI?
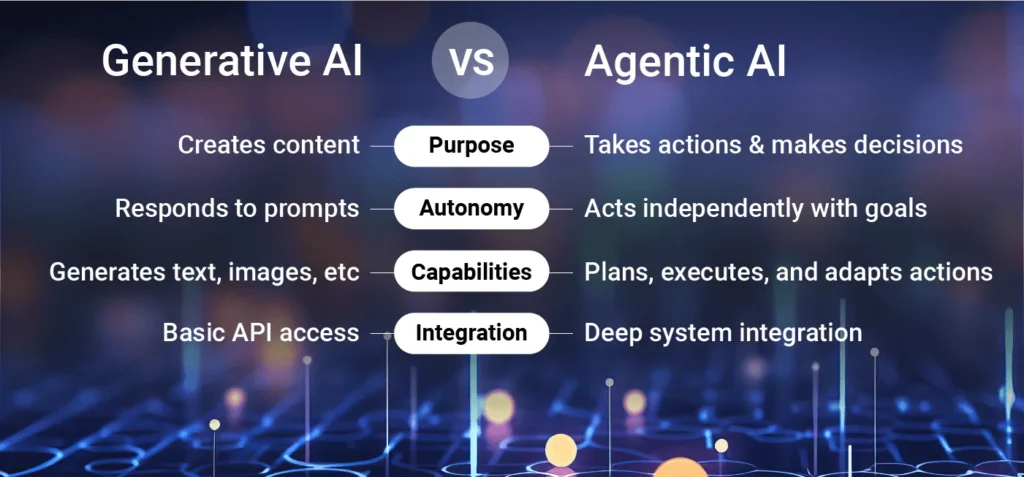
While both AI agents and generative AI fall under the umbrella of artificial intelligence, they serve distinct purposes and have different capabilities. Generative AI is primarily focused on creating new content, such as writing text, generating images, or producing videos. It uses large datasets and pre-trained models to generate outputs based on prompts.
Generative AI uses a probabilistic method to create new content by predicting the most likely next word, image, or element based on patterns learned from vast datasets. It works by calculating probabilities and selecting the most probable outcome at each step, ensuring variety and creativity in the content it generates.
On the other hand, an AI agent combines this probabilistic method with a deterministic model, which means it not only generates content but also makes decisions based on specific rules or predefined outcomes. While the generative aspect provides creativity, the deterministic model ensures the agent takes deliberate and dependable actions, such as organizing content or managing workflows, following certain criteria or instructions. This hybrid approach allows AI agents to act with both flexibility and precision. As a result, AI agents are designed to interact with other systems and execute actions based on data and instructions. They are more akin to task managers or virtual assistants within a digital ecosystem, helping to optimize workflows and drive efficiencies in real-time.
For example, while a generative AI model could write a blog post, an AI agent could help manage the content publishing process by selecting the right channels, scheduling posts, and even analyzing the performance of each piece of content. The key difference is that generative AI creates, whereas AI agents act and optimize.
Agents Will Lead to Greater Trust in AI
One of the primary concerns surrounding generative AI is its tendency to produce hallucinations—false or misleading outputs that undermine its reliability. A recent KPMG survey revealed that 60% of decision-makers cite AI hallucinations as a major concern, making it a significant barrier to adoption.
AI agents offer a solution by operating within controlled parameters and focusing on execution rather than freeform generation. The autonomous nature of AI agents is built on trust, ensuring they handle assigned tasks with precision and reliability. This trust is established through a combination of:
- Agent Instruction: Adding more configurability to the agent to help it identify the right way to respond to a request. For example, you can tell it how to select the right content to answer a question or configure it to send users to a search result page instead of just generating a natural language response.
- Performance Measurement: It’s critical to understand how well an agent is performing. This can be measured using different forms of feedback mechanisms coming directly from end users e.g. where AI generates annotations, users respond to annotations as they would respond to a human reviewer. Additionally, prompt engineers can also monitor and classify AI output. This data can be used to train AI to be more effective in future prompts.
- Self-Learning & Correction: Agents should be able to leverage user feedback for improving responses as well as identifying opportunities to automate processes or adapt strategy.
How Can AI Agents Improve DAM & Content Operations?
AI agents have the potential to revolutionize DAM, content operations, and marketing, driving efficiency, accuracy, and productivity across multiple domains. Their impact will be felt in several key areas, including:
- AI agents can help content creators, DAM admins, and librarians save significant time in metadata creation and automatically routing assets into collections and workflows based on pre-set rules
- They can help all downstream users (such as campaign or marketing teams) find the best-fit asset for each need or searches written in plain English but involving metadata filters
- Agentic AI can help go to market faster by accelerating the brand approval process with automated annotations
- They can ensure content is personalized and deployed in real-time on marketing channels based on each individual buyer’s needs and preferences
- They will also eliminate human error throughout content operations e.g. in metadata entry, content creation, brand approvals, and so on
In 2025, AI agents are going to be pretty common in martech systems including in forward-thinking SMART DAM platforms. The big challenge these vendors must deal with is to weave the capabilities of AI agents seamlessly into the daily workflows of all DAM users. In the next few sections, we will dive deeper into how AI agents will accentuate SMART DAM systems and enable content operations teams to do their work more efficiently and effectively.
AI Agents will Reduce Metadata Creation Time in the DAM
Legacy DAM systems have traditionally relied on content contributors and administrators to manually create and validate metadata. The advent of AI-powered metadata agents has already improved this process by introducing auto-suggested tags, which reduce the burden of manual tagging.
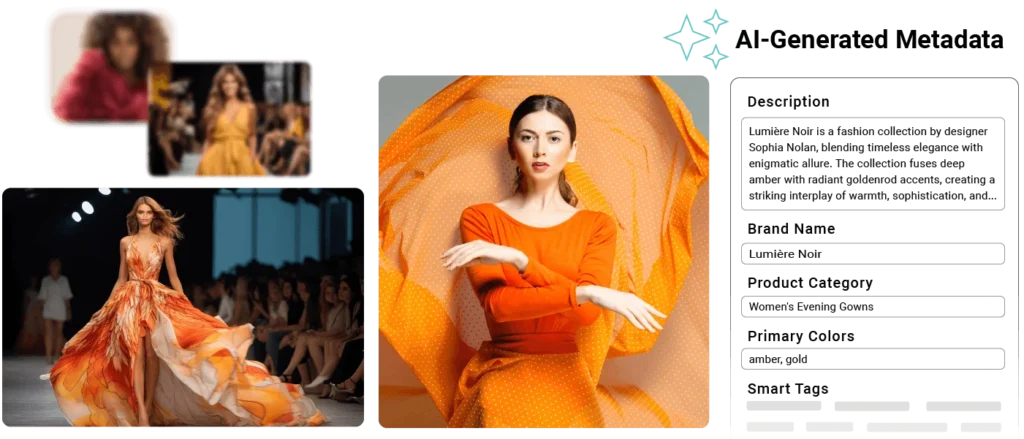
An example of what an AI Metadata agent can do: A Metadata agent can help automatically populate many more metadata fields for content such as descriptive or even dropdown fields using generative AI capabilities. It can populate metadata and organize files based on a company’s proprietary metadata model and directions provided by the DAM team. For example, if an apparel business’s agency drops a bunch of new product images, the AI agent can be trained to automatically fill out fields like brand name, product category, primary color, and even organize the relevant brands in a “Fall 2025” collection. This reduces the time a content contributor would normally spend performing all of these tasks manually. With the AI agent, assets become ready for use and search as soon as they are uploaded into the DAM.
AI Agents will Reduce Asset Search Time in the DAM
Findability is a known issue with legacy DAM systems. In 2024, 7 out of 10 Aprimo prospects named search and findability issues as the #1 reason for looking for an alternate DAM system. AI has tremendous potential to disrupt search within a DAM. AI and NLP have already enabled DAM systems to better understand user search queries and make semantic connections with assets that go beyond making simple Boolean connections.
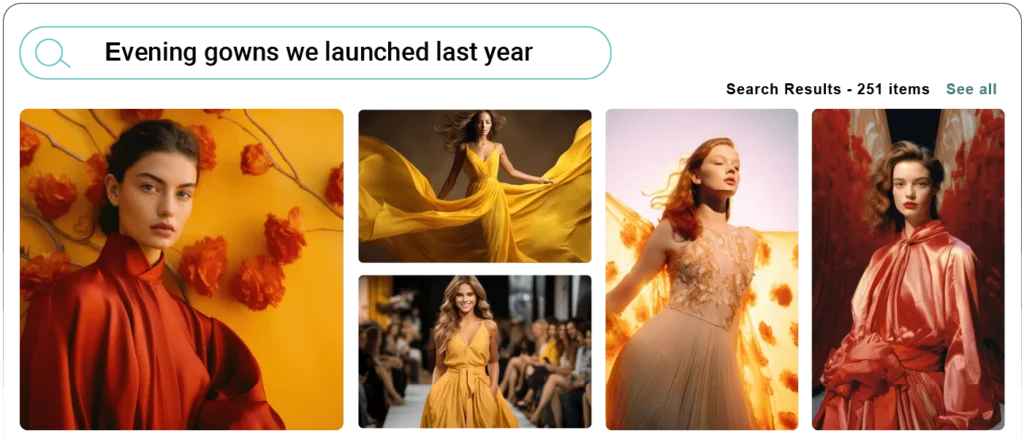
An example of what an AI Search agent can do: AI agents can help make semantic associations between search queries and relevant metadata fields to surface up better options for DAM users. As an example, “evening gowns we launched last year” will be able to filter down to collections with launch dates in the last calendar year and surface images of evening gowns from those collections only. This saves time for the user by not showing results that don’t match the said timelines e.g. an image of a product from the current year.
AI Agents will Ensure Foolproof Brand Compliance in DAM Assets
Brand and compliance workflows are an inextricable part of the DAM system. New assets – irrespective of the source – may need to be vetted by an individual, a team, or a series of individuals in a pre-determined order. Some industries like pharmaceuticals even have three separate compliance approvals required on assets (medical, legal, and regulatory). Generative AI has improved brand approvals workflows by making it easier to seek proactive feedback using AI and addressing it before a human reviews the asset.
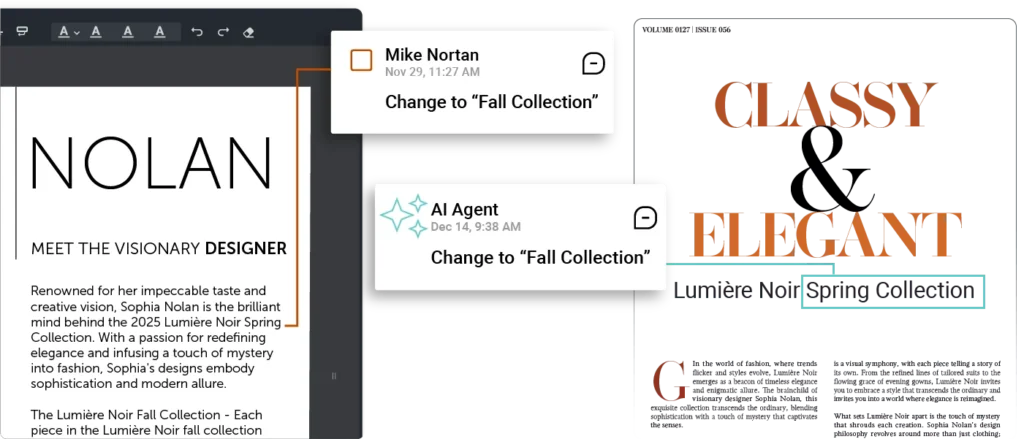
An example of what an AI Review agent can do: AI agents can enhance efficiency by providing intelligent recommendations and workflow insights, working unobtrusively in the background. Unlike task assistants, which guide users step-by-step, AI agents focus on optimizing overall productivity and addressing broader improvements. As an example, during a content review, the agent can flag previous discussions and decisions to avoid redundant conversations and approval delays.
AI Agents can Improve Customer Conversions via Marketing Personalization
Interoperability between software systems is another area where Agentic AI can help DAM systems maximize ROI for brands. Businesses have found it challenging to implement real-time, multi-channel marketing personalization as they deal with customer data in disparate data stores, restrictions on cookie use, and content sourcing challenges. When connected properly, Agents can assist in workflows in other platforms enabling brands to leverage their DAM assets for real-time content personalization.
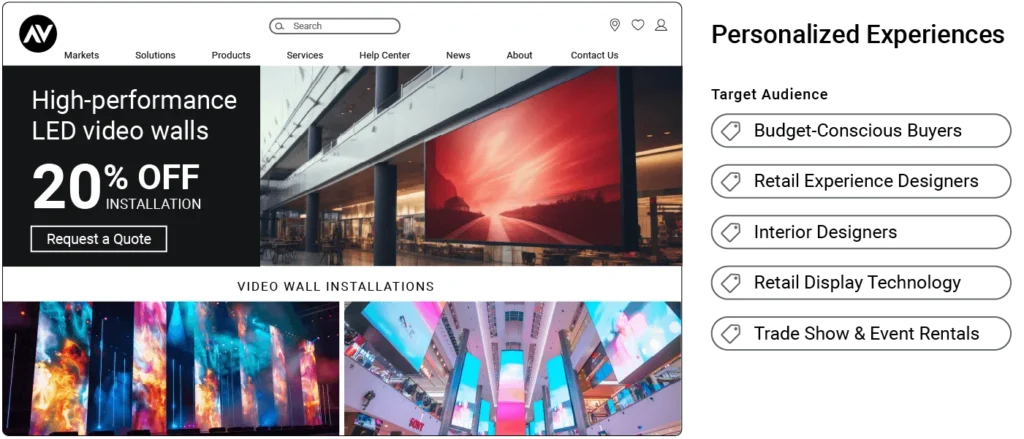
An example of what an AI Personalization agent can do: AI agents can leverage insights from the marketing channel to identify customer preferences, then source the best-fit content from the DAM, deploy generative capabilities to edit and personalize the asset and serve it to the customer – all in microseconds. For example, an AV tech website may use a personalization solution to identify a return customer with a propensity for deals. The agent could use this information to find the best images of products on sale, add the discount label on them, and push them to the homepage for the visitor in real time.
Can I Extend DAM AI Agents to Work with Other Systems?
Connecting AI with software modules, features, and systems is an integral part of having the agents make decisions and perform actions on behalf of humans. As a result, agents will have to be built on composable principles like:
- Interoperability Across Platforms: Agents on other platforms might leverage content from the DAM system to perform their tasks
- Cross-Platform Agent Utility: DAM agents could assist in workflows in other platforms e.g., complete a task in a project management system
- Extensibility: DAM agents are designed to complement, rather than constrain, the broader AI strategy
Conclusion
AI agents represent the next frontier in DAM and content operations, offering unprecedented automation, intelligence, and efficiency. As organizations prepare for 2025, integrating AI agents into content workflows will be essential for staying competitive. From enhancing metadata accuracy to accelerating content approval and enabling real-time personalization, AI agents will fundamentally reshape how businesses manage and deploy digital assets. The future of SMART DAM is not just about storage—it’s about intelligent, adaptive, and strategic content execution.